Using Predictive Analytics to Forecast Patient Volume and Staffing Needs
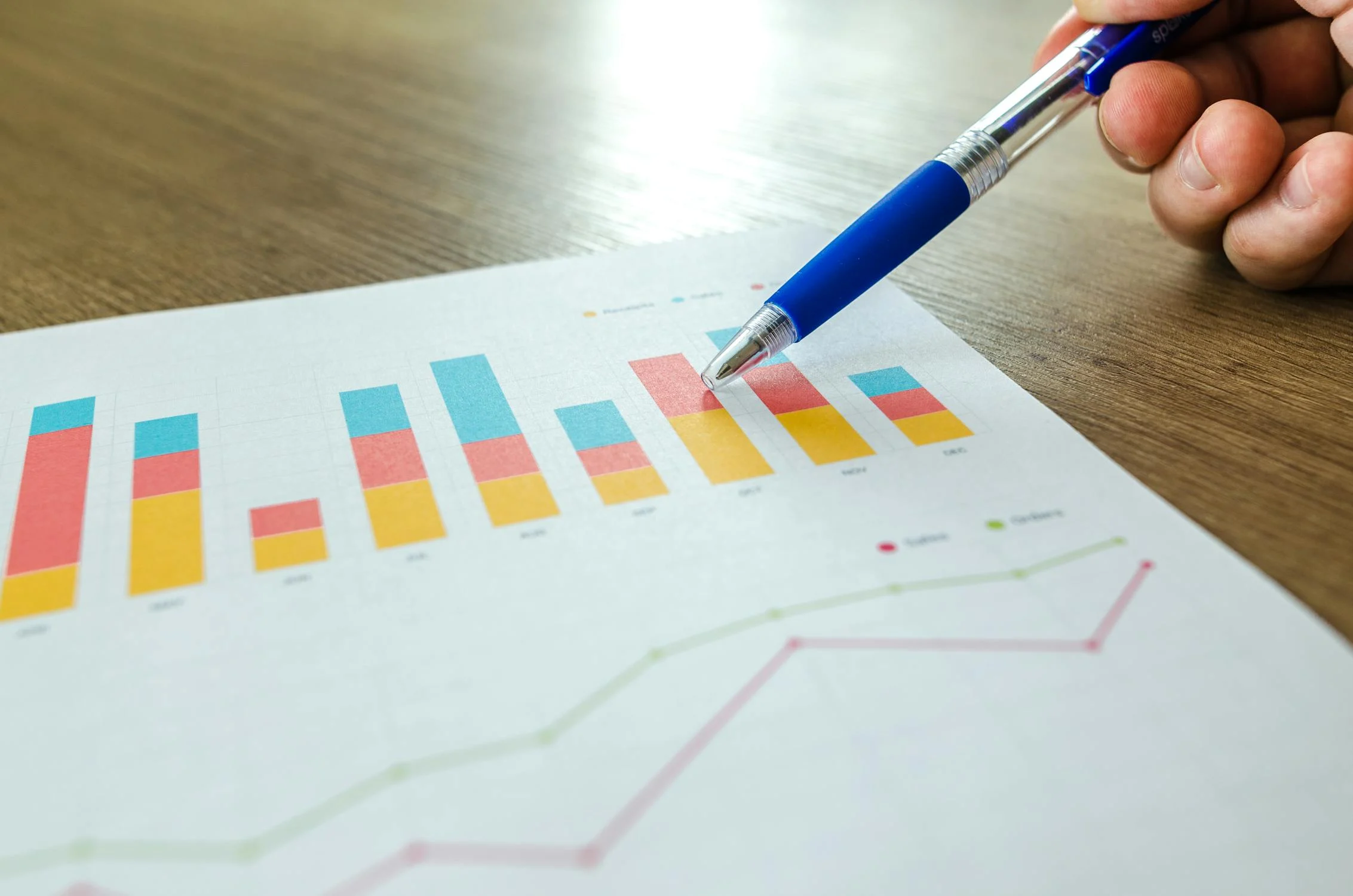
Introduction
Federally Qualified Health Centers (FQHCs) face unpredictable patient volumes and chronic staffing shortages, disrupting care delivery and straining budgets. In 2024, 65% of FQHCs reported mismatches between patient demand and staff capacity, leading to long wait times and 20% staff turnover (NACHC, 2024). Misaligned schedules increase costs and burnout, undermining quality care for underserved communities. Predictive analytics, powered by artificial intelligence (AI), offers a solution by forecasting patient volume and optimizing staffing needs with precision. AI-driven tools improve scheduling accuracy by 30-40%, cut overtime costs by 15%, and enhance patient satisfaction by 20%. Benefits include balanced workloads, financial savings, and better care access. This article explores two AI-powered features—patient volume forecasting and dynamic staffing optimization—supported by real-world examples. The result? FQHCs can align resources efficiently, ensuring sustainability and equitable care delivery.
1: Patient Volume Forecasting
A critical AI feature for FQHCs is patient volume forecasting, a process that predicts demand to guide resource allocation. Fluctuating patient visits—driven by seasonal illnesses, community events, or economic factors—make scheduling challenging. Manual estimates often lead to overstaffing (costing $50,000-$100,000 yearly) or understaffing, with 60% of FQHCs reporting patient delays (HIMSS, 2024). AI uses machine learning (ML) to analyze historical visit data, weather patterns, and social determinants of health (SDOH) like transportation access, forecasting volumes with 85% accuracy.
For example, AI can predict a 20% spike in flu-related visits in winter, allowing FQHCs to adjust schedules proactively. A 2024 McKinsey study found that AI forecasting reduced scheduling errors by 35% and improved patient access by 25%. For FQHCs serving diverse populations, models incorporating SDOH ensure equitable predictions, prioritizing high-need groups like Medicaid patients.
The people impact is significant. Administrators save hours on guesswork, focusing on strategic goals; 70% report less stress with AI tools (HFMA, 2024). Clinicians benefit from balanced workloads, reducing burnout—a key issue, with 68% of providers citing overwork (AMA, 2024). Patients experience shorter wait times, boosting satisfaction by 20%. Front-desk staff handle fewer complaints, improving morale.
The result is clear: optimized operations, cost savings, and enhanced care. Volume forecasting aligns resources with demand, cutting waste and building reserves while ensuring HRSA compliance for access.
2: Dynamic Staffing Optimization
Another transformative AI feature is dynamic staffing optimization, a process that aligns workforce capacity with predicted patient needs. FQHCs struggle to balance full-time, part-time, and contract staff, with 55% overspending on overtime or agency hires (AHA, 2024). Understaffing spikes burnout, driving turnover that costs $50,000-$100,000 per clinician. AI optimizes schedules by integrating volume forecasts with staff availability, skills, and preferences, creating real-time, adaptable plans.
For instance, AI can recommend adding a nurse practitioner for a predicted busy week while reducing hours during lulls, saving 10-15% on labor costs. A 2024 HFMA study showed AI optimization cut overtime by 20% and improved schedule adherence by 30%. For FQHCs, this ensures adequate coverage for complex cases, like chronic disease management, without financial strain.
The people benefit is substantial. Clinicians enjoy predictable schedules, with 65% reporting higher satisfaction using AI tools (HIMSS, 2024). Administrators use dashboards to monitor staffing gaps, avoiding last-minute scrambles. Frontline staff, like medical assistants, face less chaos, boosting retention—critical when 75% of FQHCs report hiring challenges (NACHC, 2024). Patients benefit from consistent care teams, improving trust and outcomes by 15%.
The outcome is compelling: reduced costs, lower turnover, and better access. Dynamic optimization ensures FQHCs deploy staff efficiently, freeing funds for services like telehealth while meeting patient needs seamlessly.
3: Real-World Examples
Real-world cases highlight AI’s impact. Unity Health Care, a Washington, D.C., FQHC serving 100,000 patients, used patient volume forecasting to address long wait times. AI predicted demand spikes, like 25% more pediatric visits in summer, enabling proactive scheduling. Wait times dropped 30%, and patient satisfaction rose 22%. Overtime costs fell $80,000 annually, and clinician burnout decreased 18%. Unity’s case shows forecasting’s role in access and savings.
In California, La Clinica de La Raza adopted dynamic staffing optimization to manage staffing shortages. AI aligned schedules with predicted volumes, cutting agency hires by 20% and saving $120,000 yearly. Staff retention improved 15%, and care continuity boosted patient outcomes—diabetes control rates rose to 68% from 60%. Administrators saved 10 hours weekly, focusing on grant applications that secured $1 million. La Clinica’s success underscores optimization’s financial and care benefits.
A Michigan FQHC network combined both AI features, targeting 40-minute wait times and 25% turnover. Volume forecasting reduced scheduling errors by 35%, and staffing optimization cut overtime by 18%, saving $150,000. Patient access improved 25%, and staff morale rose 20%. These results, backed by a 2024 NACHC report showing AI cut FQHC staffing costs by 10-20%, prove the benefits: millions saved, reduced burnout, and enhanced trust.
Conclusion
Predictive analytics transforms how FQHCs manage patient volume and staffing, cutting costs by 10-20%, reducing wait times by 25-30%, and boosting satisfaction by 20%. Patient volume forecasting aligns resources with demand, while dynamic staffing optimization ensures efficient schedules, saving $50,000-$150,000 yearly. Real-world successes—Unity’s 30% wait-time drop, La Clinica’s $1 million grant, and a Michigan network’s $150,000 savings—demonstrate AI’s power. These tools ease burnout, improve outcomes, and strengthen finances, letting FQHCs focus on their mission. As demand grows, AI-driven forecasting is essential for sustainability. FQHCs must act to harness these solutions and thrive.
Don’t let staffing chaos limit your FQHC. Evaluate your scheduling gaps today and adopt AI-driven predictive analytics to optimize resources and care. Start now for a stronger, patient-focused future.
References
- National Association of Community Health Centers (NACHC), 2024 Report
- Healthcare Information and Management Systems Society (HIMSS), 2024 Study
- Healthcare Financial Management Association (HFMA), 2024 Survey
- American Hospital Association (AHA), 2024 Report
- McKinsey & Company, 2024 Healthcare Efficiency Study
- American Medical Association (AMA), 2024 Burnout Study
No Spam —
Just Good Stuff.
Join our newsletter for actionable advice, insider knowledge, and strategies that drive real results.
No fluff, just value.
%20(1).png)