How Predictive Analytics Drives Preventative Care in FQHCs
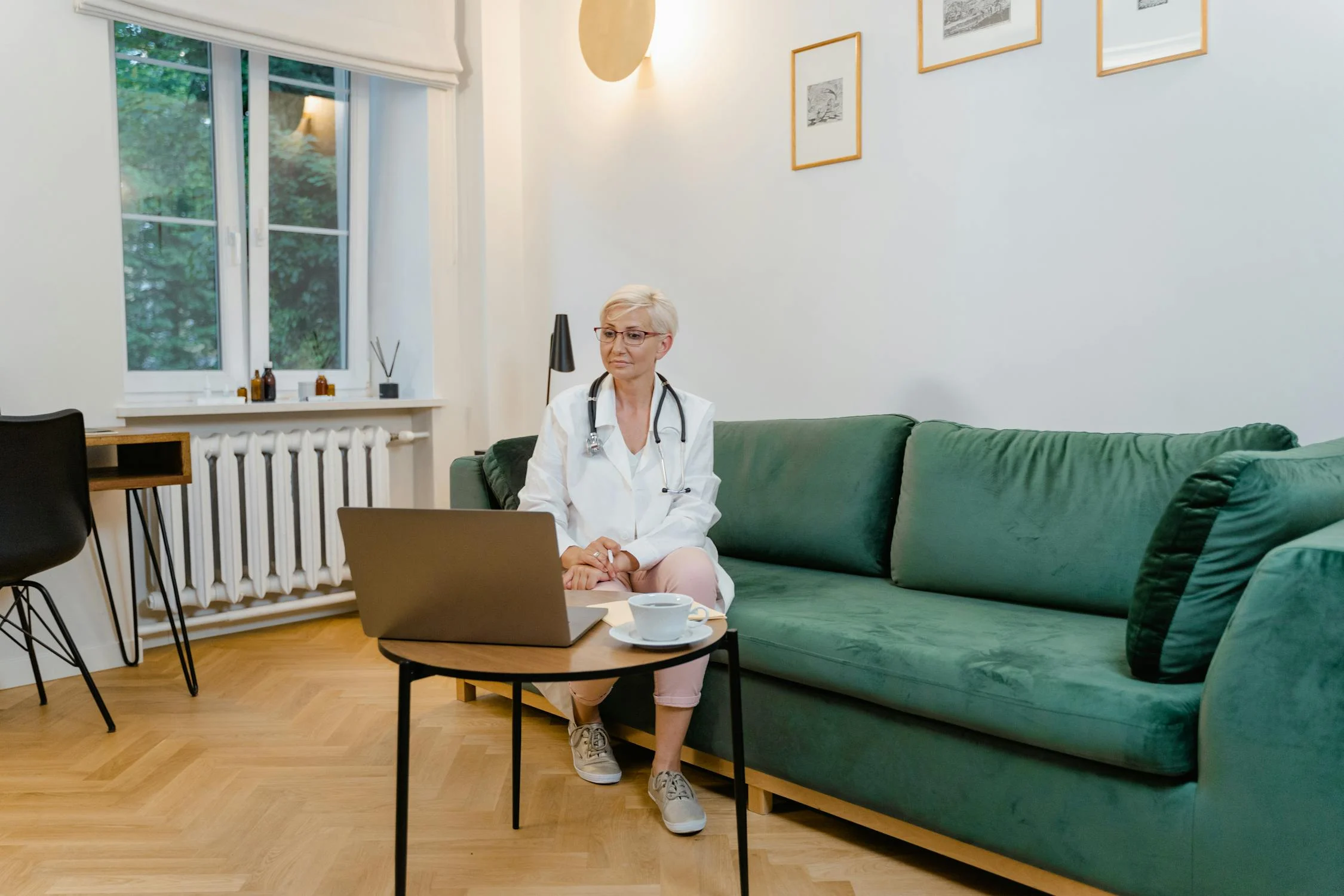
Introduction
Federally Qualified Health Centers (FQHCs) are pivotal in delivering primary care to underserved communities across the United States. These centers often grapple with limited resources while addressing complex health needs. Preventative care stands as a cornerstone in mitigating chronic diseases and reducing hospitalizations. However, identifying at-risk patients before conditions escalate remains a significant challenge.Arcadia
Predictive analytics emerges as a transformative solution, enabling FQHCs to harness data-driven insights for early intervention. By analyzing electronic health records (EHRs), social determinants of health, and other relevant data, predictive models can forecast potential health risks, allowing for timely and targeted care. This proactive approach not only enhances patient outcomes but also optimizes resource allocation and reduces healthcare costs.
This article delves into the integration of predictive analytics within FQHCs, highlighting key features, real-world applications, and the tangible benefits realized through early intervention strategies.
1: Implementing Predictive Analytics in FQHCs
Data Integration and Management
The foundation of predictive analytics lies in the effective integration and management of diverse data sources. FQHCs collect vast amounts of data, including:
- Electronic Health Records (EHRs): Clinical notes, lab results, medication history.
- Social Determinants of Health (SDOH): Housing status, employment, education, and access to transportation.
- Behavioral Health Data: Mental health assessments, substance use history.Wikipedia
By consolidating this data into a unified platform, FQHCs can create comprehensive patient profiles. Advanced analytics tools can then process this information to identify patterns and predict potential health issues.
Risk Stratification Models
Risk stratification involves categorizing patients based on their likelihood of developing specific health conditions. Predictive models utilize algorithms to assess various risk factors, enabling healthcare providers to:
- Identify High-Risk Patients: Determine individuals who may benefit from immediate intervention.
- Allocate Resources Efficiently: Prioritize care management efforts for those most in need.
- Personalize Care Plans: Develop tailored strategies addressing individual risk profiles.
For instance, a study published in Health Affairs demonstrated that predictive analytics could identify patients at risk of hospitalization, allowing for preemptive care measures that significantly reduced admission rates.medplace.com
2: Enhancing Preventative Care through Predictive Analytics
Early Detection and Intervention
Predictive analytics empowers FQHCs to detect early signs of chronic diseases such as diabetes, hypertension, and heart disease. By analyzing trends in patient data, healthcare providers can:
- Initiate Preventative Measures: Implement lifestyle interventions and medication adjustments before conditions worsen.
- Monitor Progress: Track patient responses to interventions and adjust care plans accordingly.
- Reduce Complications: Prevent the escalation of chronic conditions, thereby decreasing emergency visits and hospitalizations.
A notable example includes UnityPoint Health's use of predictive models to assess readmission risks, resulting in a 40% reduction in all-cause readmissions over 18 months. Appinventiv
Personalized Patient Engagement
Beyond clinical interventions, predictive analytics facilitates personalized patient engagement strategies. By understanding individual risk factors and health behaviors, FQHCs can:
- Tailor Communication: Send customized reminders and educational materials.
- Encourage Compliance: Promote adherence to treatment plans and follow-up appointments.
- Empower Patients: Provide tools and resources that support self-management of health conditions.
For example, CareSTL utilized AI-powered tools to identify patients likely to miss appointments, enabling targeted outreach that reduced no-show rates and improved care continuity. healthcareittoday.com
3: Real-World Applications and Outcomes
Case Study: ARcare's Analytic Transformation
ARcare, serving Arkansas and western Kentucky, implemented predictive analytics to enhance patient care. By integrating data across various platforms, they achieved:caplink.org
- Improved Diagnosis and Treatment: Enhanced accuracy in identifying patient needs.
- Operational Stability: Streamlined processes leading to better resource utilization.
- Enhanced Team-Based Care: Facilitated collaboration among healthcare providers.Wikipedia
This comprehensive approach led to improved patient outcomes and operational efficiencies.
Case Study: Healthy Planet's Predictive Modules
A large health system employed Healthy Planet's predictive modules to identify patients exhibiting early signs of heart failure. Proactive outreach by nurses resulted in a 28% reduction in emergency room visits over six months, showcasing the impact of timely interventions. LinkedIn
Case Study: Mount Sinai's Sepsis Prediction
Mount Sinai Health System utilized machine learning to detect sepsis risk 12 hours before symptom onset. This early warning system allowed for prompt treatment, significantly improving patient survival rates and reducing intensive care admissions. Medium
Conclusion
Predictive analytics stands as a transformative force in preventative care within FQHCs. By leveraging data-driven insights, these centers can proactively identify at-risk patients, tailor interventions, and optimize resource allocation. The integration of predictive models into clinical workflows not only enhances patient outcomes but also contributes to the overall efficiency and sustainability of healthcare delivery.
As healthcare continues to evolve, embracing predictive analytics will be crucial for FQHCs aiming to meet the complex needs of their communities. Investing in data infrastructure, staff training, and collaborative partnerships will be essential steps toward realizing the full potential of predictive analytics in preventative care.
FQHC leaders should initiate the integration of predictive analytics by assessing current data capabilities, exploring suitable analytic tools, and fostering a culture of data-informed decision-making. Proactive steps today will pave the way for a healthier tomorrow.
No Spam —
Just Good Stuff.
Join our newsletter for actionable advice, insider knowledge, and strategies that drive real results.
No fluff, just value.
%20(1).png)